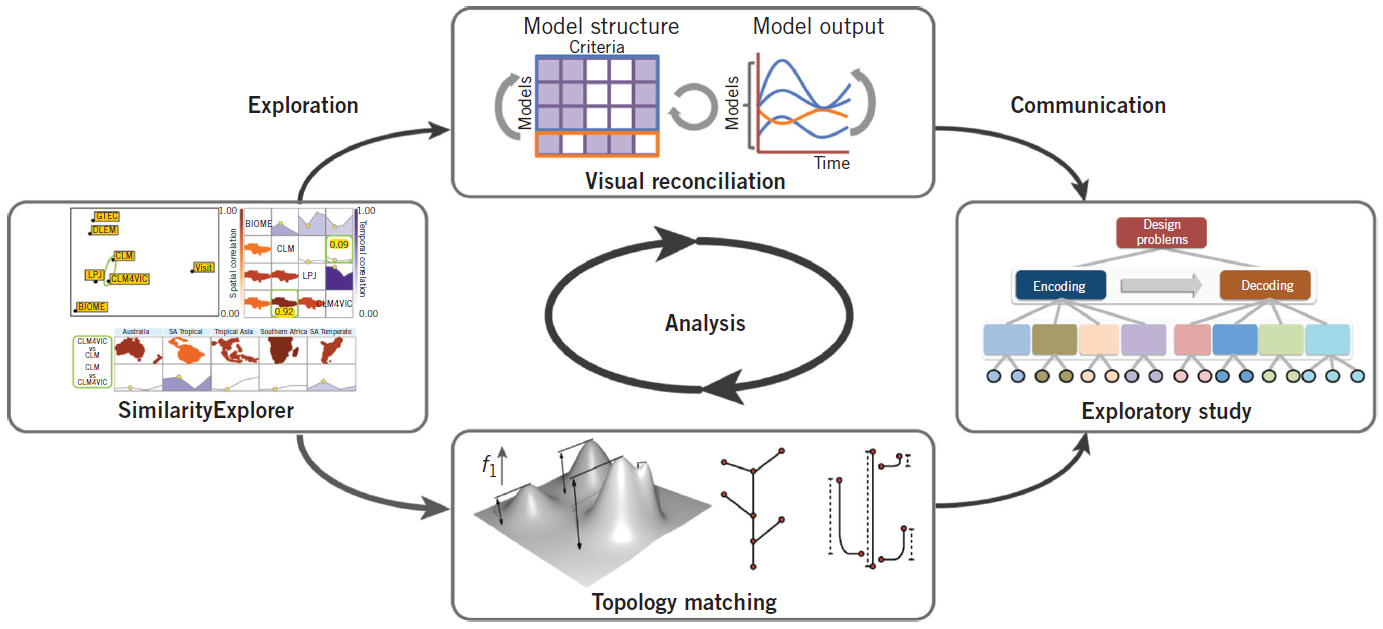
Reducing the analytical bottleneck in scientific data analysis through iterative exploration of large-scale data. In comparing (a) the state of the art and (b) a bottleneck reduction, we see the time taken for analysis and deriving insights being significantly reduced, leading to a richer exploration of alternative hypotheses on the fly, and consequently, a faster and greater return on investment of analysis time for domain scientists.
Abstract
The gap between large-scale data production rate and the rate of generation of data-driven scientific insights has led to an analytical bottleneck in scientific domains like climate, biology, and so on. This is primarily due to the lack of innovative analytical tools that can help scientists efficiently analyze and explore alternative hypotheses about the data and communicate their findings effectively to a broad audience. In this article, by reflecting on a set of successful collaborative research efforts between with a group of climate scientists and visualization researchers, the 'authors' introspect how interactive visualization can help reduce the analytical bottleneck for domain scientists.
Materials
BibTeX
@article{2016-ReducingBottleneck, title = {Reducing the Analytical Bottleneck for Domain Scientists: Lessons from a Climate Data Visualization Case Study}, author = {A. Dasgupta AND Jorge Poco AND E. Bertini AND Claudio T. Silva}, journal = {Computing in Science \& Engineering}, year = {2016}, volume = {18}, number = {1}, pages = {92--100}, url = {http://www.visualdslab.com/papers/ReducingBottleneck}, }