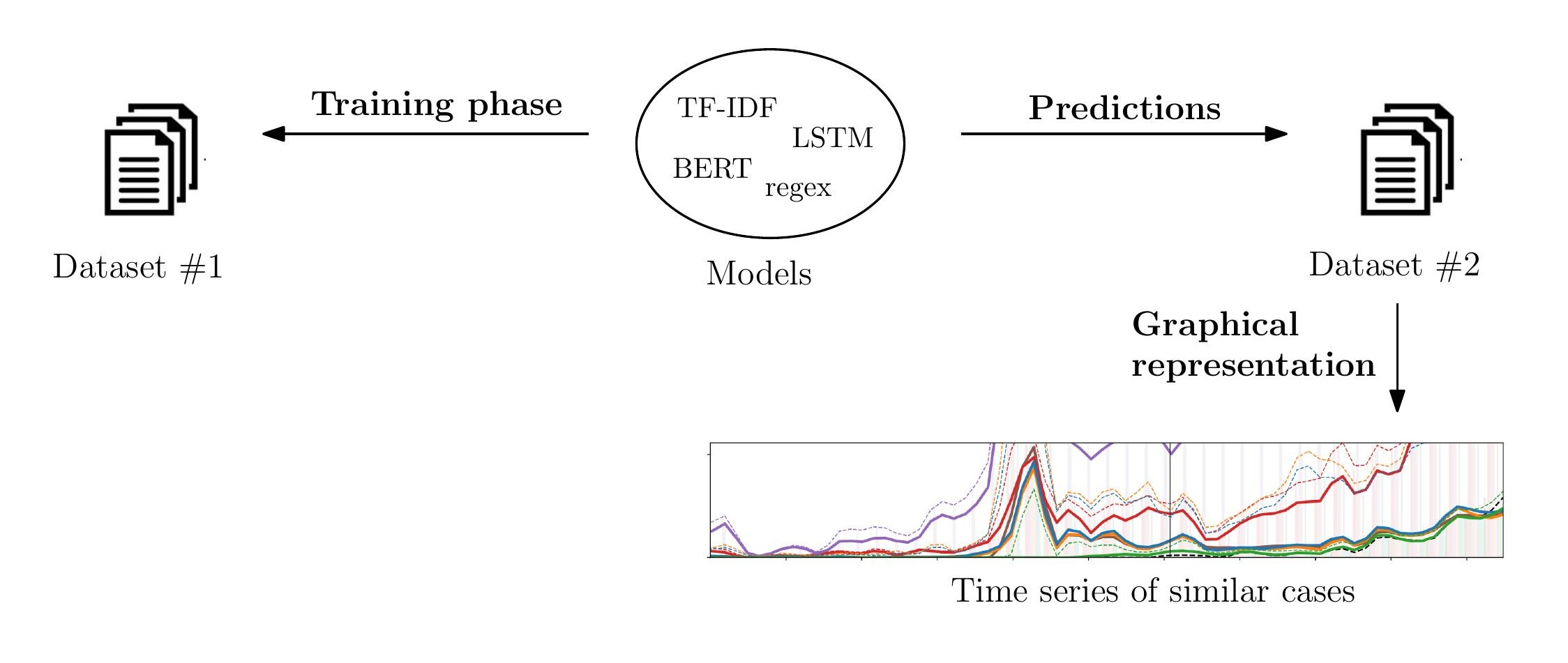
Schematic overview of the article. To understand the dynamics behind the use of a precedent, we train the models on an initial set of labeled documents, then apply these models to a larger set of data, and represent the results as a time series.
Abstract
Binding precedents (Súmulas Vinculantes) constitute a juridical instrument unique to the Brazilian legal system and whose objectives include the protection of the Federal Supreme Court against repetitive demands. Studies of the effectiveness of these instruments in decreasing the Court's exposure to similar cases, however, indicate that they tend to fail in such a direction, with some of the binding precedents seemingly creating new demands. We empirically assess the legal impact of five binding precedents, 11, 14, 17, 26 and 37, at the highest court level through their effects on the legal subjects they address. This analysis is only possible through the comparison of the Court's ruling about the precedents' themes before they are created, which means that these decisions should be detected through techniques of Similar Case Retrieval. The contributions of this article are therefore twofold: on the mathematical side, we compare the uses of different methods of Natural Language Processing -- TF-IDF, LSTM, BERT, and regex -- for Similar Case Retrieval, whereas on the legal side, we contrast the inefficiency of these binding precedents with a set of hypotheses that may justify their repeated usage. We observe that the deep learning models performed significantly worse in the specific Similar Case Retrieval task and that the reasons for binding precedents to fail in responding to repetitive demand are heterogeneous and case-dependent, making it impossible to single out a specific cause.
Materials
BibTeX
@article{2024-MLSV, title = {Empirical analysis of Binding Precedent efficiency in the Brazilian Supreme Court via Similar Case Retrieval (in-press)}, author = {Raphael Tinarrage AND Henrique Ennes AND Lucas Resck AND Lucas T. Gomes AND Jean Ponciano AND Jorge Poco}, journal = {arXiv}, year = {2024}, url = {http://www.visualdslab.com/papers/MLSV}, }