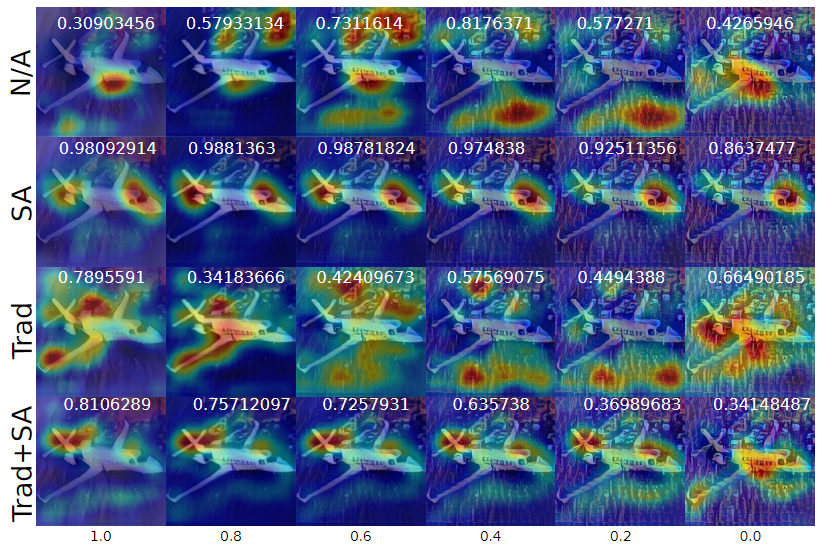
Comparison of SAM results obtained from WideResNet-101 evaluation using N/A, Trad, SA, and Trad+SA tested on the same image and style but varying the style intensity alpha as input.
Abstract
Currently, style augmentation is capturing attention due to convolutional neural networks (CNN) being strongly biased toward recognizing textures rather than shapes. Most existing styling methods either perform a low-fidelity style transfer or a weak style representation in the embedding vector. This paper outlines a style augmentation algorithm using stochastic-based sampling with noise addition for randomization improvement on a general linear transformation for style transfer. With our augmentation strategy, all models not only present incredible robustness against image stylizing but also outperform all previous methods and surpass the state-of-the-art performance for the STL-10 dataset. In addition, we present an analysis of the model interpretations under different style variations. At the same time, we compare comprehensive experiments demonstrating the performance when applied to deep neural architectures in training settings.
Materials
BibTeX
@inproceedings{2023-WSAM, title = {WSAM: Visual Explanations from Style Augmentation as Adversarial Attacker and their Influence in Image Classification}, author = {Felipe Moreno-Vera AND Edgar Medina AND Jorge Poco}, booktitle = {International Conference on Computer Vision Theory and Applications}, year = {2023}, url = {http://www.visualdslab.com/papers/WSAM}, }