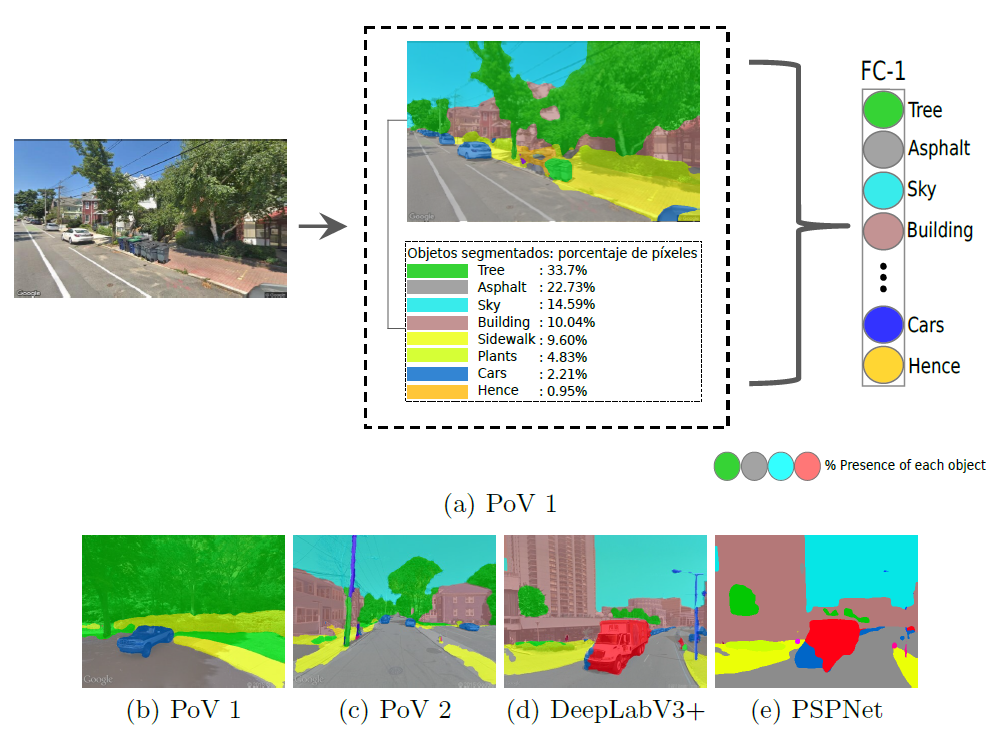
Abstract
The importance of urban perception computing is relatively growing in machine learning, particularly in related areas to Urban Planning and Urban Computing. This held of study focuses on developing systems to analyze and map discriminant characteristics that might directly impact the city's perception. In other words, it seeks to identify and extract discriminant components to define the behavior of a city's perception. This work will perform a street-level analysis to understand safety perception based on the "visual components". As our result, we present our experimental evaluation regarding the in uence and impact of those visual components on the safety criteria and further discuss how to properly choose confidence on safe or unsafe measures concerning the perceptional scores on the city street levels analysis.
Materials
BibTeX
@inproceedings{2021-UrbanPerceptionSafe, title = {Urban Perception: Can we Understand Why a Street is Safe?}, author = {Felipe Moreno-Vera AND Bahram Lavi AND Jorge Poco}, booktitle = {Mexican International Conference on Artificial Intelligence (MICAI) }, year = {2021}, url = {http://www.visualdslab.com/papers/UrbanPerceptionSafe}, }