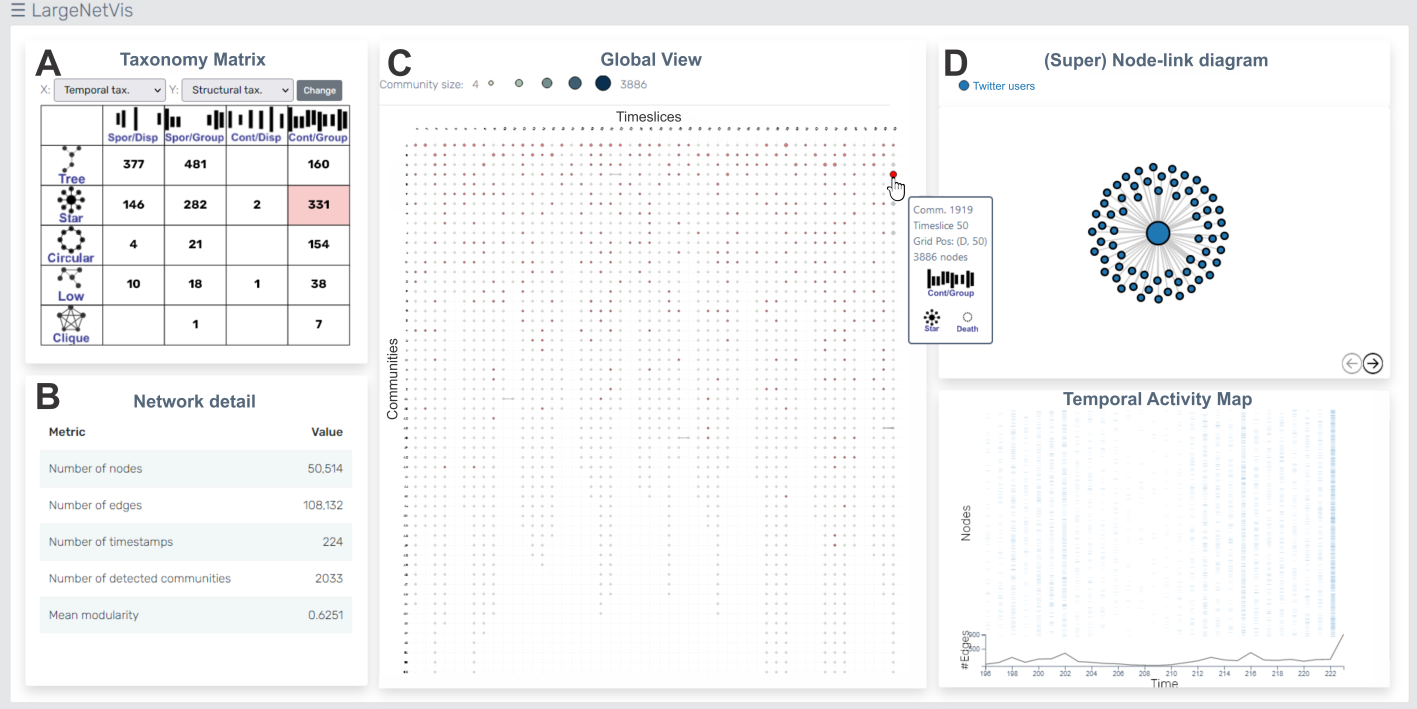
Abstract
Temporal (or time-evolving) networks are commonly used to model complex systems and the evolution of their components throughout time. Although these networks can be analyzed by different means, visual analytics stands out as an effective way for a pre-analysis before doing quantitative/statistical analyses to identify patterns, anomalies, and other behaviors in the data, thus leading to new insights and better decision-making. However, the large number of nodes, edges, and/or timestamps in many real-world networks may lead to polluted layouts that make the analysis inefficient or even infeasible. In this paper, we propose LargeNetVis, a web-based visual analytics system designed to assist in analyzing small and large temporal networks. It successfully achieves this goal by leveraging three taxonomies focused on network communities to guide the visual exploration process. The system is composed of four interactive visual components: the first (Taxonomy Matrix) presents a summary of the network characteristics, the second (Global View) gives an overview of the network evolution, the third (a node-link diagram) enables community- and node-level structural analysis, and the fourth (a Temporal Activity Map – TAM) shows the community- and node-level activity under a temporal perspective.
Materials
BibTeX
@article{2023-LargeNetVis, title = {LargeNetVis: Visual Exploration of Large Temporal Networks Based on Community Taxonomies}, author = {Claudio Douglas Linhares AND Jean Ponciano AND Diogenes S. Pedro AND Luis Enrique Correia Rocha AND Agma Traina AND Jorge Poco}, journal = {IEEE Transactions on Visualization and Computer Graphics}, year = {2023}, volume = {29}, number = {1}, pages = {203--213}, url = {http://www.visualdslab.com/papers/LargeNetVis}, }